Shining a light into the data jungle
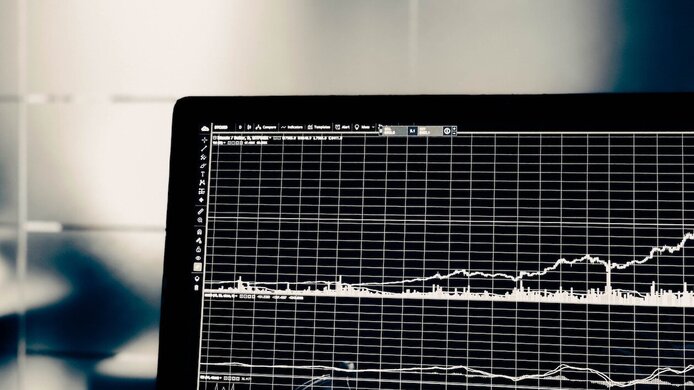
More does not necessarily equal more. This is the impression one forms when Gregor Kastner explains his field of research. “I don’t use the term big data lightly,” Kastner explains. More data does not always make us smarter; it depends on the question one needs answered. “If I keep the question simple, more data makes it easier to answer the question. But along with the amount of data, the number of questions usually increases as well. In that case it is difficult to find the aspect we are really interested in. Actually, we would need something like a treasure map.” The objective of a project by Gregor Kastner, an applied mathematician, is precisely to find out how to draw such treasure maps. The project is one of the first to be funded under the programme “Young Independent Researcher Groups” (Zukunftskollegs in German) offered by the Austrian Science Fund FWF. Aimed at early-career researchers who have recently acquired their PhD, this programme is intended to bring together different disciplines. In this case, Kastner’s project brings together five people, himself included, from the fields of statistics, machine learning, economics, social sciences and computer sciences. The group is interested in economic and sustainability issues. The corona pandemic is one of their current fields of research.
Finding relevant data
Recently one has often heard about the danger of collecting too much, rather than too little, data. But what is it that makes it difficult to have a huge amount of data and apply complex models to it? According to Kastner, an increasingly common problem is “overfitting”. This refers to the paradoxical situation that a model, in order to be credible, must not be too good. The problem is exacerbated if models are particularly flexible. In that case the model loses its informative value, comparable to a key being pressed into a soft mass. The key can be pressed into the mass not because it fits the shape, but because the mass is too soft. “In spaces of one hundred thousand dimensions, even billions of data points are useless; they get lost if the right structure is missing,” Kastner explains. It is therefore important to distinguish the relevant from the irrelevant data. Mathematically speaking, this means trying to embed a high-dimensional space in an appropriate low-dimensional one. “The simplest case would be to ignore certain data,” Kastner notes. “But there are more interesting and more effective ways.” According to Kastner, combinations of large amounts of individual data sometimes contain the same information as the data itself, but are easier to handle. The process is assisted by special preliminary assumptions, so-called prior distributions, which are specifically tailored to the problem at hand.
Number of undetected Covid cases
The current Covid-19 crisis offered Kastner's team an opportunity to test these abstract concepts. Several months ago, they tried to estimate the number of undetected infections, using Icelandic test data from April this year, because Iceland was the first country to do widespread testing and not only on people with symptoms. Kastner says the paper had only been a side-line result published at short notice, but it had attracted attention owing to the current situation. The main result, as Kastner notes, was an awareness of how high the level of uncertainty was: “We saw that you could not reliably supply an exact value; you could only give probabilities with a high degree of uncertainty, which gave rise to some frustration among media representatives.” The median number of unreported cases was 8.35 times that of the number of confirmed infections, but the range of variation depended heavily on the statistical method used. Therefore, the team emphasised the need for screenings, i.e. widely spread tests in the population, as they were subsequently carried out in Austria. In a further paper submitted for publication, the team is now investigating the economic impact of the Covid 19 crisis.
Learning with computers
One of the artificial-intelligence methods Kastner's group uses in their work is machine learning. Some of the established methods of machine learning are used today as a standard in many fields, for example for face recognition. While they can usually solve a particular problem, they are sometimes viewed critically, because they do not provide insights into the mechanisms and relationships that enable them to do so, which is also an impediment to error assessment. Kastner emphasises that his own field of statistical learning offers better opportunities in this context: “What is important for me personally: in statistical learning we extensively address uncertainty.” He notes that many of the models are also quite easy to interpret, enabling one to look into the algorithm and learn something about the system under investigation. “Forecasts are not the only issue involved here, we do data television and are able to understand what is going on in structural terms,” Kastner adds.
Speaking the same language
The special feature of the Young Independent Researcher Groups, this new FWF programme, is their interdisciplinary nature and their focus on early-career researchers between one and a maximum of five years after their PhD. There are some distinctive challenges involved, says principal investigator Kastner. “One of them is communication. We have more or less three or four areas of expertise that come together, and they all have their own terminology.” According to Kastner, it is not really that important what you call things. No-one has a problem adapting their terminology. “That said, we do identify strongly with certain terms,” Kastner notes. The fact that different disciplines have a different focus adds to the challenges. Kastner also confirms that the interdisciplinary approach has its benefits: “When it comes to application, statisticians feel blessed when they can get their hands on data from different fields.” What is crucial is to transfer statistical methods developed for a particular field to other fields. The project “High-dimensional statistical learning: New methods to advance economic and sustainability policies” is designed for a duration of four years and runs until 2023.
New opportunities for young scientists
Young Independent Researcher Groups, a programme run by the Austrian Science Fund FWF in cooperation with the Austrian Academy of Sciences ÖAW, are designed to promote excellent young researchers in Austria. Central aspects of the programme are interdisciplinarity, innovative research approaches and networking.
Personal details
Gregor Kastner is currently doing research at the Vienna University of Economics and Business. As of October 2020 he has been appointed as Professor of Statistics at the University of Klagenfurt. He is interested in Bayesian modelling of a wide variety of time series data, for instance concerning share prices. His research is concerned with both the fundamentals and the development of software tools.
Publications
Hirk, Rainer; Kastner, Gregor; Vana, Laura: Investigating the Dark Figure of COVID-19 Cases in Austria: Borrowing From the Decode Genetics Study in Iceland, in: Austrian Journal of Statistics, 49 (5), 2020
Kastner G., Huber, F.: Sparse Bayesian vector autoregressions in huge dimensions, in: Journal of Forecasting, 1-24, 2020
Gregor Kastner: Sparse Bayesian time-varying covariance estimation in many dimensions, in: Journal of Econometrics, Vol. 210, Issue 1, 2019