Is digitalization helping to make the welfare state fairer?
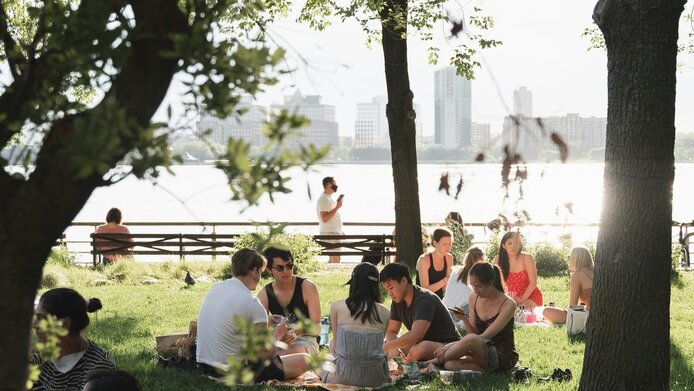
What is your idea of a modern welfare state? Technology researcher Doris Allhutter knows what most people would answer. “At the moment,” she says, “we believe that the introduction of technical systems, data and AI is the best way to modernize the welfare state. Their design, however, is often guided by ideas o administrative efficiency rather than human welfare.”
The public sector – from public employment services to the health and social security sector and local administration – is trying to ensure its services meet citizens’ needs in a more targeted way. In this context, many organizations and institutions are relying on data collection and automated processes based on new technologies. “The range of approaches is wide: simple systems that check whether application forms have been duly completed, chatbots, the development of data infrastructures or the use of AI,” explains Allhutter, who works at the Institute for Technology Assessment at the Austrian Academy of Sciences (ÖAW).
What does this mean for us citizens? This is a question Allhutter is exploring, together with European colleagues, in the research project “Automation of Welfare (AUTO-WELF)”, which is funded by the Austrian Science Fund FWF. The interdisciplinary team is conducting research on Austria and Germany, Italy and Portugal, Estonia and Poland as well as Denmark and Sweden. Each country pair represents a so-called “welfare regime”. In Austria, this regime is characterized by conservative corporatism, meaning that social benefits are strongly linked to gainful employment. Social entitlements of partners who devote their time to family and care work are often merely derivative in nature, notes Doris Allhutter.
The project “Automation of Welfare (AUTO-WELF)” explores, in the context of the European research program HERA, and supported by the FWF, the consequences of automated data systems and AI models for society.
Which systems are used where?
While the type of automated systems and their distribution vary greatly between countries and regions, the researchers still observed similar developments across Europe. “Initially, technical systems were used in individual areas: many countries tried to categorize jobseekers into groups, for example. In so doing they intended to find out what support each group needed,” says Allhutter. The algorithm that the Austrian Public Employment Service (AMS) planned to use is an interesting example. Another area of application is the attempt to use data to identify “welfare fraud”.
In the context of AUTO-WELF, the research teams explores what systems are currently being used. They zoom in on two systems per country: one in the area of core social services and one in municipal welfare, for example urban development. “Our research focuses on the way the systems work – in other words, what data are being used, how different data get interrelated and what is the intended use,” explains Allhutter.
All in the service of efficiency
Allhutter and her team conducted interviews with developers, decision-makers and case workers in Austrian public institutions, which gives them insights into the technical development, the intended benefits and possible problems in the use of the systems.
“Many systems have the objective of speeding up work processes and decisions,” says Allhutter. Rule-based systems in particular can increase the efficiency of processes, for instance by automatically checking whether application forms have been completed correctly. “However, these systems only work with a limited number of parameters.” As the researcher points out, social relationships are complex and cannot be easily modelled in computer systems. Consequently, data-based systems do not necessarily make decisions more objective and just.
Inequalities included
Another issue is the fact that data reflect social inequalities, as researchers have found out. The AMS career information system tended to suggest professions such as hairdresser or fashion retailer to women and IT to men, because the training data that the AI-based chatbot received was full of such stereotypical ascriptions. As a result, the AMS had to have the application revised. This example shows that the use of technical systems can have undesirable consequences by perpetuating inequalities.
The Dutch government's SyRI (System Risk Indication) algorithm is another, more extreme example of how technical solutions can produce “false” results. The population data it received were used, among other things, to spot families that were receiving benefits illegally. SyRI focused its checks on families with a migration background, who were then unwarrantedly obliged to pay back the benefits. Many were pushed into poverty as a result – and only learned the reason why years later.
Doris Allhutter’s take on this issue: to prevent inequalities from being exacerbated, we need a legal and institutional framework, regular system audits and the right for citizens to know what the systems do with their data.
The consequences for organizations, cities and the welfare state
“We are interested in the impact the use of these systems has. This also includes the question of how social policy changes when we collect data and design models on this basis to find out what measures work well,” explains Allhutter. The introduction of new technical systems can change institutions, for example through an increasing recruitment of people with technical skills to operate them.
Regions, cities and local authorities are also collecting data – albeit not on individuals, but on issues such as population density, climate and environmental factors. Many of them are trying to put this information to good use, notes Allhutter: “We are currently observing the trend that cities are creating so-called digital twins.” These digital twins can have positive effects: “The models can be used to spot inequalities. This can facilitate taking steps to improve the situation.” Such inequalities can be of a social nature, such as poorer air quality in certain parts of the city.
The researchers argue that in order for data-based models and technical solutions to trigger positive changes, it is vital that the individuals who are ultimately affected have a say in which areas of life the systems are used. This is one of the reasons why the research teams are holding public workshops. In Austria, people will have an opportunity to exchange ideas with the researchers in September 2024 in the context of the Ars Electronica Festival in Linz. “We want to learn what citizens really want from the welfare state and their city if we set aside the efficiency rationale,” explains Allhutter.
Next steps
AUTO-WELF will be completed by the end of October 2025. Besides the interviews with citizens, the researchers will then also have analyzed the international cases in cross-country comparison. In addition to the research papers, they will also draw up policy recommendations for the individual countries.
Allhutter emphasizes that the purported shortage of funds in welfare cannot simply be explained by increasing demand, but has to do with the way resources are distributed. So what does she think a modern welfare state should do? “It should use technical systems to achieve one thing above all: equity.”
The “Automation of Welfare (AUTO-WELF)” project runs from 2022-2025 and is receiving approximately EUR 306,000 in funding from the Austrian Science Fund FWF in the context of the European research program HERA.
About the person
Doris Allhutter is a Senior Scientist at the Institute of Technology Assessment (ITA) of the Austrian Academy of Sciences (ÖAW). She studied economics at the Vienna University of Economics and Business (WU), completed the postgraduate program “Governance in Europe” at the Institute for Advanced Studies (IHS) and gained her doctorate in political science at the University of Vienna. Doris Allhutter teaches at the Institute for Science and Technology Studies at the University of Vienna and is a member of the UNESCO Advisory Board on the Ethics of Artificial Intelligence. She has been a visiting researcher at UC Berkeley and Vassar College New York, as well as a fellow at the Paderborn Research College “Datengesellschaft” and the Digital Curation Institute at the University of Toronto.
Publications
Kaun A., Lomborg St. et al.: Crosscurrents: Welfare, in: Media, Culture & Society 2023
Allhutter D., Cech F. et al.: Algorithmic Profiling of Job Seekers in Austria: How Austerity Politics Are Made Effective, in: Frontiers in Big Data 2020
Allhutter D.: Of “Working Ontologists” and “High-quality Human Components”. The Politics of Semantic Infrastructures, in: DigitalSTS: A Field Guide for Science & Technology Studies 2019