Designing magnets for the green transition
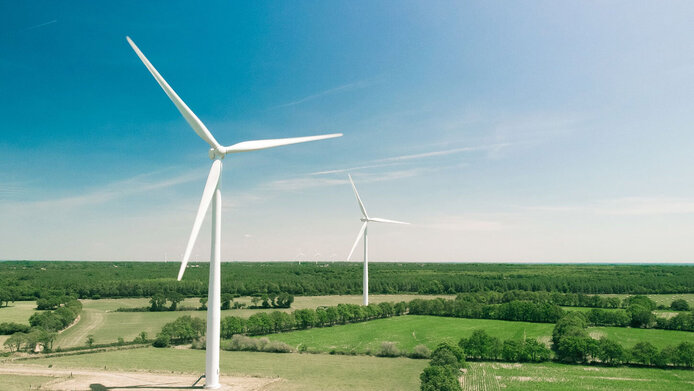
For Harald Özelt, machine learning is “the key to acceleration”. Not in the car itself, but the accelerated development of improved permanent magnets that will be used in electric cars or wind power plants. In car engines and generators, these particularly strong magnets constitute something like the silent companions of the energy transition. The interaction between a magnetic field and a current-carrying conductor converts motion into electric current (generator) and vice versa (engine). In terms of energy yield, spatial requirements and maintenance, permanent magnets are the most efficient sources of this field. They are already being used today and consist of time-tested combinations of metals and rare earths such as neodymium, terbium or dysprosium. So why does a commonly used type like the neodymium-iron-boron magnet need an update?
Minerals often contain only a very small proportion of rare earths, which makes their extraction mostly uneconomical and not very environmentally friendly. Currently, 98 percent of the rare earths needed in Europe are imported from China, and according to estimates by the EU Commission demand will quadruple by 2030. Making do with less of something that is in limited supply is therefore a crucial objective. For this reason, Harald Özelt is investigating alternative design processes that can reduce the proportion of valuable minerals required or even replace them completely. In his project, which is funded by the Austrian Science Fund FWF, the physicist is focusing on the smallest unit of strong permanent magnets, the individual magnetic grain. At the micro level, magnetic materials consist of small grains that are tightly pressed together. The objective is to optimize these grains, as well as the grain size distribution, the shape of the grains or what lies between them.
Too many options, too little time
In order to find out what composition of elements will produce more environmentally friendly, cheaper and more powerful magnets the researcher needs artificial intelligence. As Özelt explains, this is one of the simpler computation tasks: “Creating, testing and measuring magnetic grains composed of just the elements that researchers have already deemed suitable in all possible combinations is a task for generations to come.”
Özelt, who works at the Center for Modeling and Simulation at the University of Continuing Education in Krems, is therefore writing suitable machine-learning software to predict the performance parameters of the magnetic grains depending on material composition and spatial arrangement. Using powerful hardware and algorithms developed in the project, physicists and mathematicians are pursuing two goals: firstly, to investigate and better understand magnetism at the micro level, and, secondly, to design optimal permanent magnets, but also to optimize the design process itself.
More than the push of a button
Özelt and his team harness previously published measurements and their own simulations to obtain the training data for the machine-learning system. The goal is to optimize the soft magnetic and hard magnetic regions (note: the latter is where the rare earths are needed) with different compositions of the elements and variable spatial arrangement of the two phases in the grain. A magnetic field is applied to measure how “good” a magnet is at the granular level. The stronger the field needs to be to reverse the magnetization of the grain, the better.
For validation, this process is described with physical formulas in simulations. “Each conventional simulation takes one day of computing time. If we can use artificial intelligence to predict the outcome, it will only take a few seconds on each occasion. Promising predictions are then validated using physical simulation and these results are fed back into the loop.” In any case, once the model is trained, it is necessary to check its level of accuracy and the reliability of the predictions. This is done using validated test data that the model does not know.
Training plan for neural networks
A so-called neural network, in other words an algorithm that attempts to map the processes in the human brain, only faster, has already been trained with a first input batch of 4000 classical simulations. In a next step, it will be installed in an optimization framework to propose new and improved designs for magnetic grains, which will in turn be validated by classical simulation. In addition, the training methods for the neural network, i.e. the AI, are continuously being improved in collaboration with the Mathematics – Magnetism - Materials (MMM) Research Platform at the University of Vienna.
Next level magnet design
Together with doctoral student Heisam Moustafa, Harald Özelt has been working on a second FWF-funded project on magnet design since March 2023. In this project he is cooperating with the Surface and Nanostructures Research Team at Institut NEEL in Grenoble, where experimental chemical compositions of magnetic structures can be produced and measured to serve as training data. This international project is based on a neural network model for magnetism that takes advantage of the benefits of graphs. In this context, a graph describes not only the properties of individual magnetic grains, but also their interactions and mutual influence in a composite. In this way, the AI model obtains more information about the magnetic structure and can provide good predictions even with a smaller amount of training data.
The researchers also have methods “that enable us to determine why the model made a prediction and what input parameter was important for a good design,” explains Harald Özelt. By taking a “detour” via the AI, the researchers can gain new insights into magnetic phenomena and properties: “In this way, machine learning takes on the burden of discerning correlations for good magnet design in an overwhelming number of data sets and combinations.”
Harald Özelt has been a research associate at the Center for Modeling and Simulation within the Department for Integrated Sensor Systems at the University for Continuing Education in Krems since 2022. Since 2010, he has been investigating magnetism with a focus on the efficient use of rare earths in permanent magnets, machine learning and simulations of micromagnetism. Özelt acquired a PhD in solid state physics from TU Wien (2019) and studied “Industrial Simulation” at the St. Pölten Unversity of Applied Science (2012). In 2020, he spent some time doing research at the University of California (Davis/ USA). The two projects Data-driven magnet design and Design of nanocomposite magnets by machine learning are supported with ca. EUR 845,000 in funding by the Austrian Science Fund FWF.
Publication
Schaffer S., Schrefl T., Oezelt H. et al.: Physics-informed machine learning and stray field computation with application to micromagnetic energy minimization, in: Journal of Magnetism and Magnetic Materials, Vol. 576, June 2023