AI to protect our rivers
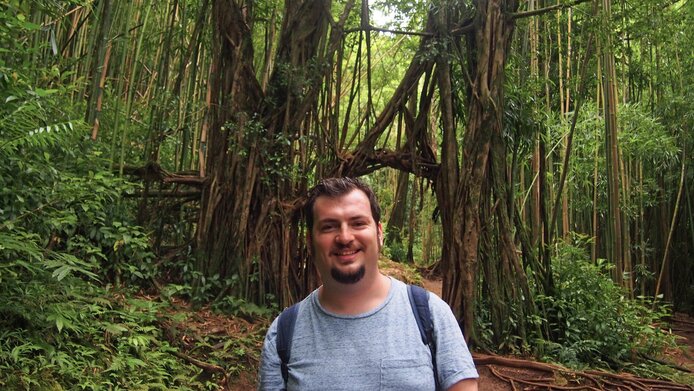
Two years ago, the banks of the River Oder revealed a gruesome sight: hundreds of fish were floating dead in the water of the German-Polish river – along with countless snails and mussels –, killed by a toxic algae bloom. The mass fish deaths were the consequence of an increased salt content in the river and the high water temperatures caused by climate change.
For a short period, environmental disasters of this kind make all the headlines. After that, attention quickly wanes again, especially when everyday ecosystems such as rivers are at issue. This is unfortunate, since it is these lifelines that are particularly affected by human activity – especially in view of the fact that many cities have developed on the banks of large rivers.
Micropollutants that constantly enter the water are particularly treacherous. While they only do so in low concentrations, they still represent a danger to humans and animals. In order to track down these pollutants, computer scientist Atakan Aral and his research team from the University of Vienna are using AI. In this interview, the computer scientist explains how the project, which is funded by the Austrian Science Fund FWF, can contribute to sustainable water protection.
World Water Day is celebrated annually on March 22 and organized by the United Nations. This year's motto is "Leveraging Water for Peace".
Mr. Aral, many people are aware that rivers are polluted with substances such as fertilizers or plastic. Micropollutants, on the other hand, are not very well known. What are they?
Aral: Micropollutants is the name experts use to refer to various chemicals which are very difficult to detect in water, because they only occur in low concentrations and are also colorless and odorless. This is worrying, because it means that these compounds can go unnoticed for a long time when they find their way into bodies of water. The danger is twofold: on the one hand it is their inconspicuousness, and, on the other, the sometimes serious effects they can produce. These substances can be highly toxic, carcinogenic or hormonally active, or they can contribute to antibiotic resistance. We are talking about flame retardants, pharmaceutical compounds, combustion by-products, preservatives and many other man-made chemicals.
None of this should be found in rivers. But how do these substances get into the water?
Aral: One of the sources are factories when they use river water either indirectly to cool their plants or directly for industrial processes. If there is a failure in the water treatment plant they use to discharge the water back into the river, certain pollutants will escape continuously – and unnoticed – over a longer period of time. However, micropollutants are also washed from the surface into watercourses, for example in heavy rainfalls, or they seep into the groundwater from landfill sites and eventually end up in rivers. This means that almost every river surrounded by human activity is contaminated with micropollutants. Of course, some rivers are so heavily polluted at the macroscopic level that micropollutants are not at the center of attention.
In the context of your research project you have found new ways to monitor micropollutants with the help of AI. Where did the data come from that you use to train the AI model?
Aral: Our team carried out measurements on two rivers, the Ergene River, and the Kokemäenjoki River in Finland. The former in particular, which is situated on the border between Greece and Turkey, is heavily polluted by the textile industry, which makes it an interesting case of usage. We obtain direct data on micropollutants from water samples that are analyzed in the laboratory. Unfortunately, this is a costly undertaking and cannot be done in real time. That's why it was part of our research project to develop detectors that can monitor the flow live. For this we used artificial intelligence, too, to draw conclusions about micropollutant contamination from factors such as water turbidity or color, which we can observe in real time. In addition, the AI enables us to extrapolate from the selectively collected laboratory data to the overall situation.
Detectors, water samples, laboratory analyses – that sounds like a complex infrastructure to generate the volumes of data required for AI. Can this be manageable?
Aral: For sure, the necessary infrastructure should be as sustainable as possible. We don't want the river to be flooded with sensors, especially as these devices are battery-powered and usually remain in the environment after their useful life. That is why placing sensors everywhere is not a good way to get the high-quality data we need for our AI models. Our goal was to use AI to make generalizations about the entire river network from measurements collected at a few spots. We were recently able to show that fifteen sensors are enough to cover a very large area in the northwest of Turkey, which previously required seventy sensors. We used AI to analyze the sensor data and make an intelligent selection of critical sensor locations.
How did you manage that?
Aral: Our AI model is a neural graph network consisting of many nodes. The connections between these nodes indicate how strongly changes to a parameter in one node affect other nodes. The collected data is then used to create a digital representation of the river course, whereby we take into account the hydrology of the river. For this task we rely on experts. After the AI's independent learning process these experts decide whether the connections between the nodes really make sense. This network ultimately enables us to trace changes from a ramification to the source. Fingerprinting is also important in this context: we have identified micropollutants that are characteristic of certain industrial sectors, which we can then trace back to their place of origin.
Unlike human fingerprints, these pollutant markers can change if, for instance, the same factory uses different chemicals on different days. How do you cope with that?
Aral: We can deal with that, because our learning process is not something that happens once and then stops. Our AI model keeps learning continuously from the periodically extracted measurements and data measured in real time. As a result, the connections between nodes can weaken or change over time. The system improves itself and adapts to changing conditions. Experts refer to this as online learning. However, this is not only necessary because of the pollutant fingerprints; climate change is also causing changes, for example in the concentration of micropollutants.
The terrain is yet another challenge: you have to monitor some challenging river sections, where power supply and internet access are scarce, both of which are essential for AI. Doesn't that limit the areas where your method works to highly developed industrialized countries?
Aral: It is precisely because we are observing very remote areas that we have also optimized the way in which data is collected. Along rivers, for instance, mobile phone networks are sometimes not available. So one can either build cable ducts and radio masts, or find cheap, sometimes even environmentally friendly solutions to get the data. We have tried various things in this respect, for instance working with drones that regularly fly to these locations. But we have also developed methods of conducting machine-learning directly at the source. Normally, the data for the learning process is sent to cloud storage. If the processing of the raw data and the initial learning steps take place on the spot, much less data needs to be transferred. This decentralized learning is one of the main results of our project.
What other areas of application for this method come to mind?
Aral: Not needing a cloud for data processing has several advantages, one being that you don’t have to transmit personal information. So if the AI models of the well-known text and image generators could run directly on our end-devices to some extent, this would be a benefit in terms of data protection. Rural regions are another area of application for this method, since connectivity there is often very limited. By starting the learning process on the end-devices, the amount of data to be transmitted is drastically reduced, which means that even remote areas could benefit from AI. We have also had to develop particularly energy-efficient algorithms for our detectors, which are very handy wherever power supply is scarce. Again, countless possible applications are conceivable, not least after natural disasters, where emergency services could use AI for image or video recognition.
Atakan Aral studied computer science and engineering at the Technical Universities of Milan and Istanbul, where he received his doctorate in computer engineering. Today, he is a postdoctoral researcher at the Scientific Computing Research Group of the Faculty of Computer Science at the University of Vienna. His research interests include resource management for edge computing, edge AI and the Internet of Things, with a focus on environmental monitoring systems.
As coordinator of the international SWAIN-Projekt, Aral also has a leading role in the TROCI-Projekt (start 2024), which is funded by the EU and the Austrian Science Fund FWF. He is a member of the Environment and Climate Research Hub (ECH) at the University of Vienna and chairs the Climate Change Group of the Center for Artificial Intelligence and Machine Learning (CAIML) at TU Wien.
The research project SWAIN
Industrial plants use river water for various purposes, which can result in micropollutants being discharged into the water. These substances have a serious impact on aquatic ecosystems and humans. In the context of the FWF-funded project “Sustainable Watershed Management Through IoT-driven Artificial Intelligence (SWAIN)”, principal investigator Atakan Aral and his interdisciplinary and international team developed an AI-based monitoring system for micropollutants.
In addition to the University of Vienna, the research consortium includes TU Wien, the Università della Svizzera Italiana in Switzerland, TU Istanbul, Bogazici University and the Finnish Environment Institute. AI-placed sensors are used to collect the data required for machine learning. Human expertise is incorporated into the AI's continuous learning process, allowing conclusions to be drawn about micropollutant levels based on data collected in real time. The project also receives funding from Switzerland, Turkey and Finland through the CHIST-ERA network.