When metals become brittle like glass
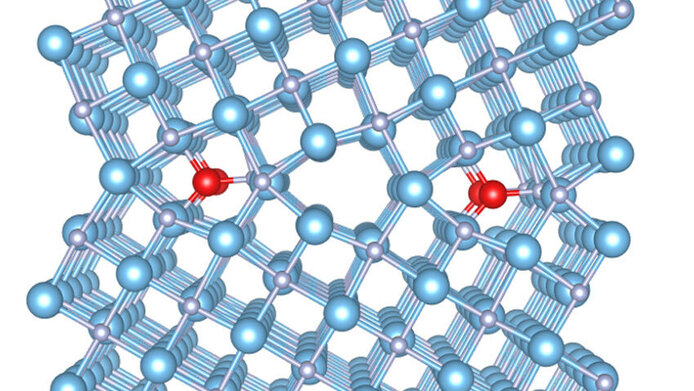
This research involves enormous computational effort, with individual calculations keeping supercomputers busy for days on end. The objective is a description of the behaviour of metals, especially of the processes in their inner structures, at the grain boundaries. Metallurgy is a research field of great relevance for all industries that work with metals and electronic elements, such as metal manufacturers, the automotive industry, chemical companies or recycling facilities. So far, however, the complexity of the calculations has been a stumbling block. In a project funded by ASMET, the Austrian Society for Metallurgy and Materials, in cooperation with the Austrian Science Fund FWF, the materials scientist Lorenz Romaner from the University of Leoben is trying to circumvent this problem using machine learning methods. His approach allows him to replace time-consuming physical calculations and to correct errors in the simulations.
Imperfect crystal lattices
“Grain boundaries exist in almost all crystalline materials. There are cases of perfectly regular crystals being produced, such as single crystal silicon in the semiconductor industry, but most structure-giving materials are polycrystalline and involve different size grains,” Romaner explains. Between these grains, within which the lattice is regular, there are boundaries where the crystal structure changes. “Depending on the material, these grains are a few nanometres to hundreds of micrometres in size” – that is, between a few tenths and a few millionths of a millimetre.
These grain boundaries have a major impact on the properties of materials, especially when the materials are alloys of different elements. “Many phenomena in the material occur precisely at the grain boundary, such as brittle fracture. It is possible for materials that are normally malleable and easy to process to suddenly become brittle.” Romaner describes the example of liquid gallium being dripped on to aluminium: “The gallium runs into the grain boundaries and in these places the aluminium breaks like glass.” The structural weakness of metals is only one among many aspects, albeit a negative one. Interactions at the grain boundaries can also achieve many desirable effects, such as avoiding brittle fractures or making certain electronic applications possible.
Simulating quantum effects
The mathematical tool that researchers like Lorenz Romaner use to calculate such effects is called density functional theory, an approximation method for complex quantum mechanical processes such as the ones occurring at grain boundaries. Using this tool requires an enormous effort. “It involves atomic-level simulations that we use to look at how alloying elements behave at the grain boundary. Basically, we want to understand how the energy changes when we shift alloying elements from the interior to the grain boundary,” Romaner explains.
Usually, this leads to an energy gain because alloying elements do not fit perfectly into a crystal lattice of another element, and moving an atom of such an alloying element from the inside of the crystal to the grain boundary has favourable energetic consequences. This energy quantity, called segregation energy, is what Romaner's group is computing. But the large amount of computation the process requires is an impediment. It seems actually possible to reduce the effort, however, because many of the calculations are quite similar, which begs the question whether they have to be carried out with the highest level of effort each and every time.
Artificial intelligence
It is exactly this kind of situation where the use of machine learning suggests itself. “If I feed the right information to an algorithm, it may be able to predict further energies very efficiently,” is how Romaner describes the goal of the project launched in June 2021. He reports that initial tests are promising: “We can see that this works very well. A large number of atomic calculations can be replaced by training algorithms and using what they produce to get a complete overview of different segregation energies.”
Correcting errors
But Romaner has more ambitious goals. Despite the high effort involved in the density functional theory calculations, they sometimes produce faulty results because it is an approximation method. Again, Romaner wants to use machine learning to correct these errors. He intends to make the algorithms remember, in a manner of speaking, where density functional theory produces errors and adjust the results accordingly. “This will become relevant in the second phase of the project,” Romaner predicts. “We are currently in the process of building a database to this end.” In this context, the researchers in Leoben are exchanging ideas with various partners worldwide.
The related experiments are carried out by Romaner's colleague Ronald Schnitzer, also a researcher at the University of Leoben. The researchers use a so-called atom probe, which can examine materials down to the size scales of individual atoms and identify interesting reactions very quickly. “We have started to contact other groups to create a larger database relating to the investigation of segregation energies with atom probes. This is work in progress and will enable us to train models on the basis of these data in the future,” says Romaner, and adds, “The training of density functional theory makes a lot of sense if I have a large number of calculations and a clever algorithm. The question I ask myself is why this wasn't done much earlier.”
Personal details
Lorenz Romaner studied physics in Graz and has been Professor of Metallurgy at the University of Leoben since 2020. He is interested in the computer-aided description and simulation of materials to describe their electronic, magnetic or mechanical properties. The project “Hybrid modelling of grain boundary segregation” (2021–2024) is funded by the ASMET Research Award with EUR 300,000. This award is sponsored by the Association of the Metal Producing and Processing Industry and has been given every three years in cooperation with the FWF since 2017.
Publications
Dösinger C., Spitaler T., Reichmann A. et al.: Applications of Data Driven Methods in Computational Materials Design, in: Berg- und Hüttenmännische Monatshefte 167, 29–35, 2022
Scheiber D., Romaner L., Pippan R., Puschnig P.: Impact of solute-solute interactions on grain boundary segregation and cohesion in molybdenum, in: Physical Review Materials 2, 2018
Leitner K., Scheiber D., Jakob S. et al.: How grain boundary chemistry controls the fracture mode of molybdenum, in: Materials & Design, Vol. 142, 2018