New method for age determination
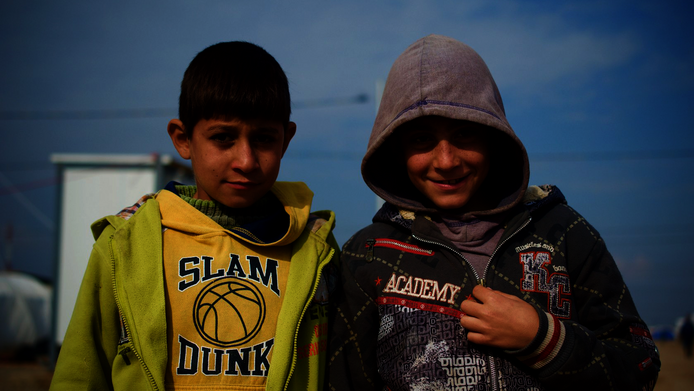
Determining the age of young people as accurately as possible has become a highly political issue in recent years. Underage refugees enjoy special protection in Austria and Germany, but the authorities often do not have access to the necessary documents to verify the age of the asylum seekers. In such a case, the authorities conduct interviews, and a person’s age can also be estimated using X-rays and computer tomography images. The use of X-rays on healthy people, however, is controversial in ethical terms. While there is a legal exception in Austria which permits the taking of X-rays to determine the age of healthy individuals in the context of asylum procedures, there is no such law e.g. in Germany. As part of a project financed by the Austrian Science Fund FWF, the team succeeded in developing automated age determination without X-rays on the basis of magnetic resonance imaging.
X-ray as the standard method
“Age determination using X-ray images was developed in the 1950s,” explains the principal investigator Martin Urschler. “The method is based on the growth of bones, for instance the finger bones. In young people, these elongated bones consist of two parts with cartilage in between. This area is called the growth joint. When the person is fully grown, the joint closes. The farther away a bone is from the body, the sooner this process is completed.” This is not the same for every human being; there is biological variability caused by genetic predisposition or elements of lifestyle, such as nutrition. “The standard method today is to take X-rays of the hand, wisdom teeth and a computer tomography image of the collarbone. The collarbone is close to the body; it closes its growth joint later than the hand bones and thus supplements the data from the hand. The teeth, on the other hand, develop from a different germ layer than the bones, and their development is relatively independent of nutrition, which provides complementary information about dental development,” says Urschler. The actual estimation is carried out by trained radiologists and dentists together with forensic experts. “In practice, it is a matter of estimating a minimum age in order to determine whether someone is highly likely to be a minor or not. In case of doubt, the decision is made in favour of minority.”
Controversial method
As Urschler explains, the use of X-rays is currently the standard method, but it is controversial: “On the one hand, there are health concerns, especially because of computer tomography of the collarbone, where many important organs are affected by strong X-rays. On the other hand, it is known that the estimates can diverge between six months and two years, depending on the expertise of the people making the estimates.” Urschler's team was therefore looking for a new method of age determination based on magnetic resonance imaging (MRI) which additionally uses machine learning methods to solve the existing problems.
Automatic age determination
“The FWF project follows up on a study we launched in 2011. We were looking for volunteers between the ages of 13 and 23 who would agree to have MRI pictures taken of their hands, clavicles and teeth for a small fee. At present, this database contains almost 350 individuals, all of them male and of Caucasian descent, in order to exclude ethnic variability for the time being.” As part of the FWF project, the team has now developed an algorithm that automatically estimates the age of these data sets. “We trained the software on one part of the data and tested the estimates on the rest of the database,” explains Urschler. In order to do this, the computer had to learn to identify autonomously the relevant bones in the MR image. For this purpose, methods of machine learning were also used, which Urschler's group developed for general medical application together with the Graz University of Technology.
High accuracy
It was found that the computer achieves similar accuracy in age determination to that achieved by forensic experts. The method was also able to keep up with larger studies based on the examination of X-ray images. “We hoped that we would be able to significantly improve the accuracy of age estimation using the MRI method and machine learning. We could not achieve that, however, we are in the range of the X-ray method, but we have the advantages of improved objectivity, reproducibility and the lack of radiation exposure.” Mean deviation of the chronological age determined by the computer from the real chronological age was one year. A more accurate error estimate will be published soon. The project ran for three years and was completed at the end of 2018. Urschler emphasises that there is no database of X-ray images for machine learning comparable to the MRI database created in Graz. The analysis of MRI images would therefore be the method of choice for the future. But in order to make the new method feasible in practice, further research on other ethnic groups and on women is required. “Then the method would legally be the lowest-impact means of age determination and would have the potential to replace current X-ray methods thanks to its comparable accuracy,” notes Urschler.
Personal details Martin Urschler is a computer scientist and is conducting research at the University of Auckland in New Zealand. Before that, he worked on a project at the Ludwig Boltzmann Institute for Clinical Forensic Imaging in Graz, whose term ends in 2019. His research interests lie in computer vision, particularly medical image analysis with possible applications in clinical and, more particularly, in forensic medicine. Publications