Machines learn to be curious
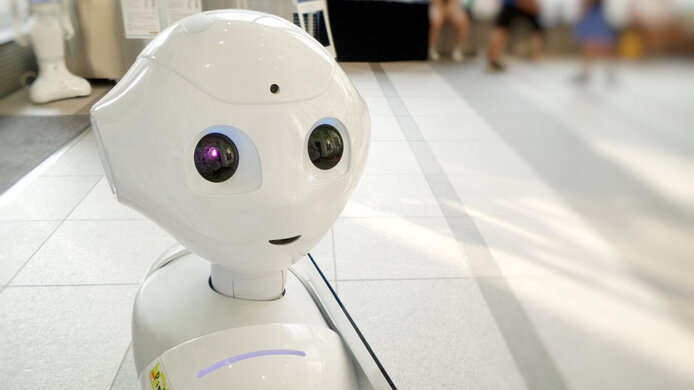
Machines are acknowledged for their reliability in doing exactly what they were built for. Unlike people, however, they are quickly out of their depth in situations where qualities such as independent thinking or curiosity are required. This is why researchers around the world, including Austria, are trying to expand the capabilities of robots. A group led by the robotics expert Markus Vincze from the Vienna University of Technology (TU Wien) tried to create machines that can respond to unfamiliar objects. In a project financed by the Austrian Science Fund FWF, robots were taught to recognise when they are unfamiliar with something and then proceed to acquire the missing information from the Internet.
The human model
“We took our cue from human beings,” explains Markus Vincze. “When people don’t know something, they set out to look for information – in books in days gone by, and today mostly on the Internet. The idea was to have robots do the same” While modern-day robots are able to identify objects in camera images by comparing them against an internal database, the machines are still quite helpless when confronted with objects unknown to them. A new approach was needed. The keyword in this context is “deep learning” – learning from large amounts of data.
3D perception
“The first step in recognising an object is segmentation,” Vincze explains. This involves making a distinction between objects and their background, such as a coffee cup and the table on which it sits. “There are methods that do work well for stand-alone objects,” notes the researcher. The next step is figuring out what objects one is dealing with. “This can be difficult, for instance, when objects partly overlap and defy exact delineation.” Once an object has been recognised, the aim is to create a 3D model of it so that the robot can reach for it and pick it up. This type of three-dimensional perception comes very naturally to humans, but again represents a challenge for machines, says Vincze: “Small children can do this from the first year of life, they perceive objects as three-dimensional.” All these methods have now been implemented in robots in the context of a three-year basic research project along with international partners.
Becoming aware of ignorance autonomously
Vincze's group was interested in the situation where a robot fails to recognise an object, such as the coffee cup on the table. First of all, the researchers needed to establish criteria for the machine to decide whether it has recognised an object or not. “The robot compares a photo of the object against a database. By means of statistical methods it decides to what extent the observed object resembles objects in the database,” explains Vincze. “This results in a certain value. If the value is too low, the robot should proceed to take a picture of the object and search the Internet.” The team implemented this by using various search algorithms, including ImageNet and the standard Google image search. Then Vincze's group analysed which nouns appear most frequently in the texts accompanying the images. In order to improve the results, a counter-check was carried out: The resulting term was searched again on the Internet and the images thus found were compared with the image of the unknown object. This improved the quality of the search.
HOBBIT prototype
These new approaches were tested with prototypes. Vincze's team and international project partners in Italy, France and the UK used robots developed in earlier research projects for the tests. One of them was HOBBIT, a machine designed for use in old people's homes for chores such as finding things that have been mislaid. For a practice test, the team used an office environment with ten typical utensils lying on a desk: keyboard, mouse, hole punch, stapler, and so on. The objects were all known to the system. For the test, one of the objects was removed from the database, and the robot had to identify the unknown object.
Context makes all the difference
In this setting, Vincze and his team explored to what extent the context affects successful recognition. If, for example, most of the objects on a table are tableware, there is a heightened probability that the unknown object also belongs to this category. “Such contextual information can be analysed and used in a targeted manner and thus narrow down the search,” says Vincze. In that way, the results keep improving. The researcher stresses that this was a basic research project and that a long way remains before robots become truly independent: “Humans still have to intervene frequently.” Vincze predicts that it will take decades until robots will be able to develop a degree of autonomy similar to that of humans – but a start has been made.
Personal details Markus Vincze is a roboticist and a researcher at TU Wien, where he heads the Vision for Robotics laboratory that he set up in 1996. He is particularly interested in methods of visual perception for robots in real-life environments.
Publications