Human-centred artificial intelligence
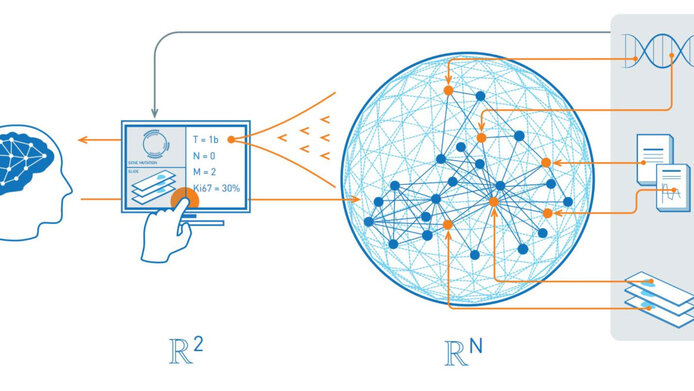
“There used to be this joke,” says Andreas Holzinger. “You go to see your bank advisor for a loan. The advisor looks at his computer screen and says he can’t approve your loan. When you ask why, he replies: because the computer says so.” This is how Holzinger circumscribes a problem that has been around for a long time, but has recently taken a back seat owing to the great successes of artificial intelligence (AI) through neural networks. Algorithms in cars already recognise traffic signs, mobile phones understand our language, and translation tools deliver amazingly accurate results – and all of this happens completely autonomously, without human involvement. In certain areas of application, however – medicine being one of them – this “fully automated” black-box approach is raising more and more legal and ethical issues. In a project funded by the Austrian Science Fund FWF, Andreas Holzinger is exploring how humans can once again become part of the decision-making process.
Including human experience and understanding
Holzinger says that the problems are home-made: “Almost exactly ten years ago, I gave a lecture at Carnegie Mellon University in the US, a top university in the field of AI, where I put forward the idea of adding human intervention to AI applications.” Holzinger was disappointed, however, by how little resonance this received from the research community: “At the time, they wanted to make everything fully automatic – without any human involvement.” Over time, neural networks became more and more powerful but also more and more complex, and thus more “opaque”. The reason is that they are not coded by humans, but learn autonomously from huge amounts of data. This approach has the side effect that in most cases it is no longer easy to understand exactly how the algorithms arrive at their decisions.
The EU’s new data protection directives are now causing AI specialists to rethink the approach. “The General Data Protection Regulation contains something like a right to explainability. So if an autonomous AI does not approve your loan, you have the right to ask about the underlying criteria on which this decision is based,” says Holzinger. For AI developers this is a problem, however, because the research community has so far mainly worked on algorithms for automatic and autonomous decisions and was not really prepared for this.
The concept of “Explainable Artificial Intelligence” promises a solution, as it is designed to make AI decisions more transparent. Intensive research is currently being devoted to this area. One variant of explainable AI is implementing the possibility to ask “if-then” questions. This requires making so-called counterfactual statements, i.e. fictitious “what-if” assumptions designed to question decision-making processes. Holzinger notes that such interventions require interactive user interfaces, and for these you need to have an idea of how good the explanations of decision-making processes are.
How good is the explanation?
“This is one of the focus areas of our work. We have developed the concept of causability, which goes beyond pure technical explainability,” Holzinger reports. Our concern is not only providing the technically relevant aspects of decisions, but also assessing their quality. According to Holzinger, causability, which may be paraphrased as “identifiability of causes”, describes the measurable extent to which an explanation helps human experts to understand in a specific context of use. There cannot be one “universal explanation” for everyone. In order to enable conceptual understanding as a function of individual prior knowledge, for instance, explanations must also be adaptable, which results in a need for further user interfaces.
Two of the fields in which Holzinger and his team apply the knowledge gained are medical or biological topics, where explainability of the results is of particular importance. The researcher hastens to underline the general character of his research within the framework of this basic research project. While biology and medicine are important areas of application, he explains that “the results are also applicable to agriculture and forestry, climate research or even zoology.” Holzinger not only expects better insight into AI techniques, but he also holds out the prospect that it may enable them to provide more robust results in the longer term, to be less biased and also to need smaller quantities of data for training. “Our work is generic, which is why it is being so well received internationally,” Holzinger notes.
Human beings at the centre
Holzinger does not venture to make a prediction of how AI will develop in the future and how neural networks may change our everyday lives, but he does express a wish: he hopes that a holistic approach to artificial intelligence will be found that has humans at its centre, and that computer methods not only include abilities such as contextual understanding – which Holzinger calls “common sense” –, but also social, ethical and legal aspects. All of this should be assembled under the notion of “Human-Centred AI”, which he coined, with the aim of reconciling artificial intelligence with human values, ethical principles and legal requirements.
Personal details
Andreas Holzinger is a computer scientist and head of the Human-Centered AI Lab at the Medical University of Graz. Since 2019, he has been Visiting Professor of Explainable AI at the Alberta Machine Intelligence Institute in Edmonton, Canada and has recently become fellow of the International Federation of Information Processing (IFIP) . The project A Reference Model of Explainable AI for the Medical Domain, which started in 2019, is scheduled to run for four years and is receiving EUR 393,000 in funding from the Austrian Science Fund FWF.
Publications
Pfeifer B., Secic A., Saranti A., Holzinger A.: GNN-SubNet: disease subnetwork detection with explainable Graph Neural Networks, in: bioRxiv (Preprint) 2022
Holzinger A., Malle B., Saranti A., Pfeifer B.: Towards Multi-Modal Causability with Graph Neural Networks enabling Information Fusion for explainable AI, in: Information Fusion, Vol. 71, 2021
Holzinger A., Carrington A., Mueller H.: Measuring the Quality of Explanations: The System Causability Scale (SCS). Comparing Human and Machine Explanations, in: KI – Künstliche Intelligenz, Vol. 34, 2020