Artificial intelligence reveals gender bias
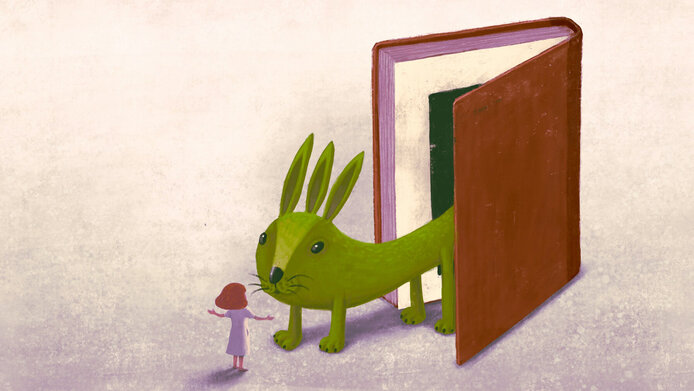
Alice’s Adventures in Wonderland is one of the best-known children's books in world literature to this very day. The book by author Lewis Carroll was first published in the UK in 1865 under the rule of Queen Victoria. This was a time when the roles of men and women were still strictly defined. Women were associated with family, motherhood and raising children. Their place was the home, while men explored the wider world.
In Carroll’s book, the girl Alice seems to have little time for these role models. Filled with a keen sense of curiosity, she enters the magical Wonderland where she encounters unusual characters and communities. Fearlessly, Alice ventures forth into domains that are really reserved for men. The fact that the heroine defies gender stereotypes was unusual then – but not only then. Even today, a majority of children's books simply reflect social norms and gender stereotypes. This diagnosis is confirmed by research, including one study from 2021, which analyzed children's books from the years 1960 to 2020 with a focus on gender roles. One of the key findings was that male protagonists are still in the majority.
AI scans children's books
The problem with this state of affairs is that stories have a formative influence on young readers. It has been proven that gender stereotypes develop early on in childhood. The impact this has on the emotional and cognitive development of children and young people becomes manifest, inter alia, in school performance and career choices.
The statisticians Camilla Damian and Laura Vana-Gür set out to create more awareness of this misrepresentation that becomes firmly ensconced at an early age. They developed a model that uses artificial intelligence (AI) to scan children's literature for gender bias. On the basis of numerous gender-related aspects, which the small team specifically defined in advance, the software was trained to analyze texts in order to produce a gender representation score for each book. In theory, language processing programs are already capable of such tasks – just think of the capabilities of ChatGPT. However, it takes more to obtain a truly valid result that can be understood and used as a basis for recommendations.
Computers learn implicit gender inequalities
“Current algorithms for language processing have the disadvantage of embracing bias they find,” explains Vana-Gür. In her FWF-funded 1000 Ideas project, this young researcher wanted to exclude such an assimilation process. In order to protect the analysis itself from gender bias from the outset – and not in retrospect, as is usually the case – the researchers fine-tuned the training process for the algorithms by means of time-consuming manual adjustments. The steps taken included a literature review based on findings from sociological, psychological and educational research in order to define indicators of gender stereotypes.
The researchers themselves then analyzed children's literature to determine factors that can be implicitly associated with gender bias. They looked at the fields of work of the various characters and also explored whether they were portrayed on the basis of their appearance or rather their intelligence. Other character traits that were relevant to the analysis include friendliness and aggressiveness, which are typically reserved for female and male characters, respectively, notes Vana-Gür. In addition, the researchers conducted data visualizations and created scores for centrality and influence to show in what way the main protagonists are connected to the supporting characters.
Project website
Achieving explainable results
The research team developed the measurement method on the basis of this qualitative evaluation and comprehensive data collection. They applied the method to around 30 children's books from those listed in Project Gutenberg – which primarily covered classic children’s stories such as Alice’s Adventures in Wonderland, Cinderella, Aladdin and Hansel and Gretel - works that are no longer subject to copyright and were therefore freely accessible to the researchers.
“This systematic approach has great potential, although it is very complex,” notes Vana-Gür, in reference to another advantageous factor that is becoming increasingly important in AI research: “The results must be transparent and understandable, which is an important goal in the project.” Researchers refer to this as “explainable AI”. For the researchers, explainable measurement outcomes are the prerequisite for being able to provide valid guidelines that can raise awareness of problematic under- and misrepresentations of gender in children's literature. “We consider it important to obtain an interpretable overall assessment in order to make it possible for publishers, educators and parents to make well-founded decisions,” says Gür.
In order to further establish the new tool, there is a need for more training and additional databases for children's literature to serve as a testing ground. Vana-Gür emphasizes that they are just at the beginning. Ideally, the automatically produced gender score will ultimately help to come upon fewer stories on the children's book market that tell of charming princesses and bold astronauts. After all, the world of boys and girls, and, ultimately, the world of us adults, has a great deal more to offer, as Alice already successfully demonstrated 150 years ago.
Personal details
Laura Vana-Gür studied economics in Romania and acquired her doctorate in statistics at the Vienna University of Economics and Business (WU). In 2021 she was appointed assistant professor in the Computational Statistics research area at TU Wien. Prior to that, she held the position of university assistant (postdoc) at WU Vienna. Her research focuses on the development of statistical methods and software that enable the analysis of complex data, especially in the field of sustainability.