Artificial intelligence could improve therapy for lymphoma
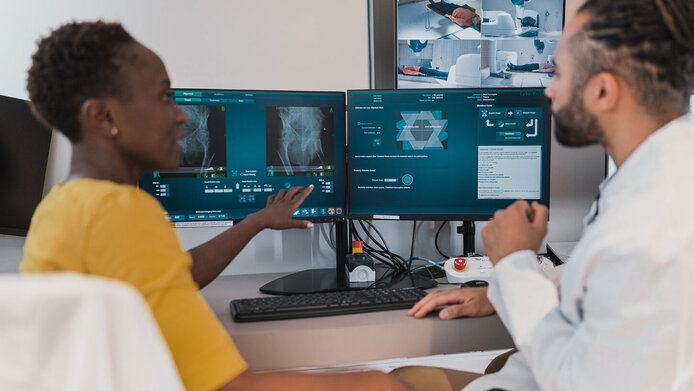
It is particularly young people that are affected by Hodgkin's lymphoma. While this form of cancer, which develops in the lymph glands, is particularly aggressive and can spread quickly, it comes with good chances of a cure. Treatment, however, is often accompanied by serious short-term and long-term side effects. The standard treatment is chemotherapy, but patients affected by certain subtypes of this lymphoma also need to undergo radiation therapy.
Researchers at the Nuclear Medicine Division of the Medical University of Vienna at the Vienna General Hospital (AKH) are now looking for new ways to improve diagnostics. Co-funded by the Austrian Science Fund FWF, the three-year project HOLY-2020: Individualized Therapy in Hodgkin Lymphoma has them working with European partners on a new method that combines imaging medicine with artificial intelligence.
“The diagnostic methods currently used to predict the course of the disease are relatively crude and inaccurate. We are using artificial intelligence algorithms to improve the accuracy of predictions,” explains Alexander Haug, deputy head of the Clinical Department of Nuclear Medicine. One of the benefits of the new diagnostic tool could be to provide clarity earlier on about whether aggressive radiotherapy is required or whether chemotherapy is sufficient, thereby increasing the certainty that patients receive adequate treatment.
Nuclear medicine visualizes tumors
The current gold standard of diagnostics for Hodgkin's lymphoma can map the cancer cells in the body with high accuracy. This approach uses a combination of positron emission tomography (PET) and computed tomography (CT). Patients are administered very weakly radioactively labelled glucose which is absorbed by the body's cells. Tumor cells have higher energy requirements and quickly absorb a lot more of these labelled sugar molecules than normal body cells.
PET scans show a precise distribution of radioactive sugar molecules, thereby mapping the size and shape of the lymphoma. By combining them with CT images, which provide a 3D image of the inside of the body, one can pinpoint the position of the cancer cells in the body and thus determine very precisely how far the cancer has progressed in the body. The degree to which individual regions are affected provides indications on risk factors and chances of cure.
Haug and his team assume that the PET and CT images contain even more information than is gleaned by existing evaluations. For instance, the data show the distribution of intensities within the lymphomas. At some sites, a much higher numbers of sugar molecules are measured than at others. The researchers’ analyses have revealed dozens of different parameters that can be distinguished on the basis of this heterogeneous distribution. “Even the trained eye cannot draw any conclusions about disease progression from these patterns,” says Haug. “But we are confident that artificial intelligence will be able to use it to predict prognosis.”
Training based on existing patient data
In order to develop an appropriate AI system, the team analyzes the PET/CT images and links them to the course of the disease in the respective patients. This is then fed to the self-learning algorithms as training data. The AI thus learns which of the patterns that lie hidden in the images are associated with good or bad prognoses. When confronted with new image data that was not part of the training, the AI tool should eventually be able to provide new prognostic assessments. In medical science, this type of interpretation of image features in radiology is also known as radiomics.
In this project, Haug and his colleagues at the Medical University of Vienna are collaborating with research institutes in Paris and Barcelona. Overall, the researchers have already collected more than 200 data sets on different disease courses in Hodgkin's lymphoma. Initial AI analyses are now underway. “Data harmonization is a particular challenge with many radiomics solutions,” notes Haug. “Images from different scanners differ in details. Color shading, for instance, is always slightly different from one scanner to the next. First we must standardize such properties in a time-consuming process.”
Set to run until fall 2023, the project aims to confirm that this kind of AI diagnostics is essentially possible. In subsequent research, the approach could then be further developed with a view to practical application.
Personal details
Alexander Haug has been Associate Professor and Deputy Head of the Clinical Department of Nuclear Medicine of the Medical University of Vienna at Vienna General Hospital (AKH) since 2013. He also heads the Christian Doppler Laboratory for Applied Metabolomics located at the AKH. Before moving to Vienna, he worked at the Großhadern University Hospital in Munich. The European project “HOLY-2020” (2020-2023) is receiving EUR 196,000 in funding from the Austrian Science Fund FWF.
Publications
Krajnc D., Spielvogel CP., Grahovac M. et al.: Automated data preparation for in vivo tumor characterization with machine learning, in: Frontiers in Oncology, Oct. 2022
Zhao M., Kluge K., Papp L. et al.: Multi-lesion radiomics of PET/CT for non-invasive survival stratification and histologic tumor risk profiling in patients with lung adenocarcinoma, in: European Radiology, Oct. 2022
Leithner D., Schöder H., Haug A. et al.: Impact of ComBat Harmonization on PET Radiomics-Based Tissue Classification: A Dual-Center PET/MRI and PET/CT Study, in: Journal of Nuclear Medicine, Oct. 2022
Berzaczy D., Haug A., Staber PB. et al.: RECIL versus Lugano for Treatment Response Assessment in FDG-Avid Non-Hodgkin Lymphomas: A Head-to-Head Comparison in 54 Patients, in: Cancers (Basel), Dec. 2019